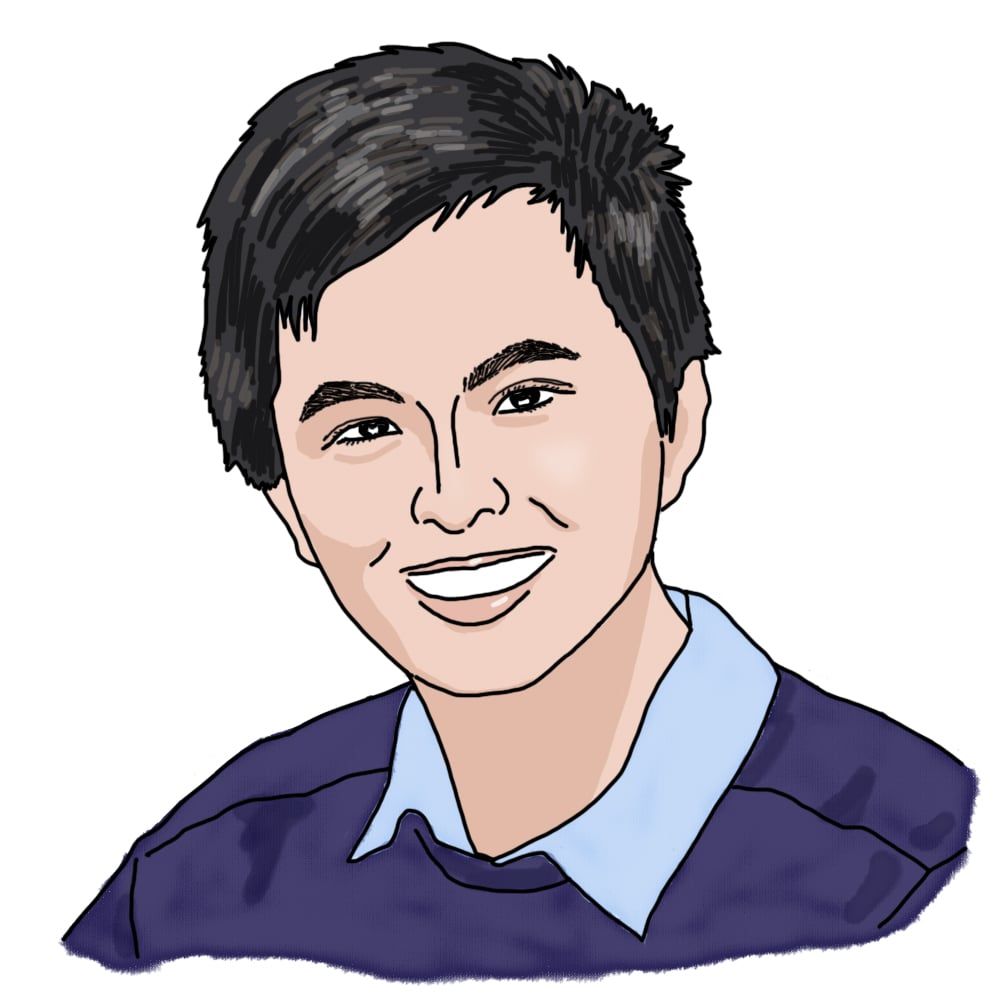
I think I am slowly going insane by Andi Q. '25
This is your brain on AI-generated videos
Last week, Professor Han made an exciting announcement during 6.5940 (TinyML) lecture: the class was holding a competition, with AI development boards (worth $500+) as the top prizes! The competition involved watching hundreds of AI-generated videos and rating how realistic each looked. His lab was trying to create a benchmark for evaluating AI-generated videos, and outsourcing to MIT students was the best way to get the high-quality human-labeled data that OpenAI can only dream of.
The first-place prize was an NVIDIA Jetson Orin Nano, which Professor Han had demo-ed at the start of the semester running a ChatGPT-like application on a retro CRT-looking01 The same type of display you'd normally see on old-timey electronics like analog oscilloscopes display. It was so cute, and I really wanted to build one for myself, so I knew I had to compete.

It’s so cute! I want one :)
The dataset consisted of a few thousand videos generated by a handful of AI video models from prompts like “The archer launches the arrow towards the target” and “The robotic arm slides a towel across the table”.
“Wow, these videos… all look terrible” was my immediate reaction after watching and labeling a few of them. The AI models were bad – hilariously bad in many cases. I vividly remember watching one video generated from the prompt “Soccer players in purple celebrate as the crowd cheers” which just showed purple people-looking blobs jiggling on a blurry green background and one of them morphing into the soccer ball. It was nothing like the sleek videos OpenAI likes to tout in its demos.
(This is the kind of slop that these AI models would typically generate. To be fair, all the videos were generated for free, so I didn’t expect them to be that good in the first place.)
Yet one random Chinese AI model – “minimax” – was exceptionally good. Not only were its videos smooth and crisp, but they usually also followed the laws of physics. When I first saw a minimax video, I thought it was a real video mixed in as a sanity check for labelers.
(This video is taken from minimax’s website. I didn’t feel like sifting through the actual dataset for a minimax video, but most of them looked good like this.)
Two days and a few hundred videos in, I began picking up on some interesting trends. Each model would fail in predictable ways: OpenSora would always generate people as Lego mini-figures, CogVideoX would follow prompts very well but produce grainy/jittery videos, and minimax (my beloved) would simply not fail. (Each model (even the really awful ones) was also really good at generating videos of photorealistic feet for some reason02 They were probably trained on videos found on the internet , which I found funny.)
Eventually, my brain just went on autopilot, labeling video after video for an hour. It was a good break from my usual MIT schoolwork, and it was somewhat entertaining seeing all the ways the AI models could hallucinate cars and people morphing into other things.
But that’s when everything started to go downhill.
It turns out (unsurprisingly) that minimax is not perfect. However, it only ever fails in extremely subtle ways, like a strand of hair experiencing a tad too much gravity or a tree branch swaying a tad too little in a gust of wind. Without me realizing it, these subtle flaws began to mess with my mind.
When I took a break from labeling videos to eat dinner in the dining hall, I caught myself staring at some ice cubes swirling around a friend’s drink.
“Hang on,” I thought, “is that how liquids should behave in real life? Those ice cubes seem to be moving around a little too fast.”
In fact, liquids do behave that way because I was experiencing real life and not some AI-generated pseudo-reality. Still, it was unnervingly disorienting to look at those ice cubes.
I still really wanted that NVIDIA board though, so I just shrugged it off as me being tired (it was a Thursday evening, after all), went to sleep, and continued labeling videos the next day. With each new video I labeled though, real life became ever so slightly less realistic. Human hair seemed to disobey the laws of gravity (somehow the videos had primed me to expect hair to act like it does in Pixar movies). Car tires seemed to rotate too quickly for the speed they were traveling at (that was another thing none of the AI models could get quite right). Sometimes I’d just stand on the sidewalk and watch the trees swaying gently in the wind, wondering if my eyes were just playing tricks on me or if the leaves were truly morphing into those strange, unnatural shapes.
I finally decided to stop on Sunday after I had labeled over 1500 videos. Unfortunately, this wasn’t quite enough to win the NVIDIA board – another student had labeled 700 more videos than me. Instead, I won a Qualcomm Snapdragon Development Kit – less cool than the NVIDIA board03 But somehow also twice as expensive to buy but, as Professor Han assured me, still plenty powerful for running a large language model. All for the low, low price of my sanity.
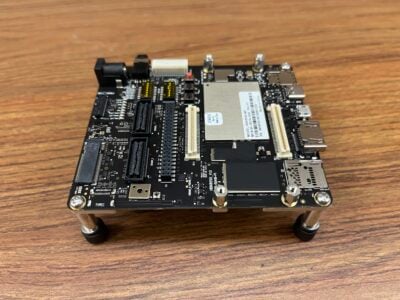
Honestly, this was worth the effort I spent labeling all those videos.
Shortly after receiving the kit, I got an email from Scale AI04 A big data labeling tech startup that I used to intern at about participating in a “fun coding challenge” that was clearly just another data labeling task for benchmarking a large language model they had developed. I think I’ve learned my lesson about labeling AI-generated content though, so no thanks, Scale. (Maybe next time though, if you’re offering me a free GPU as a prize.)
- The same type of display you'd normally see on old-timey electronics like analog oscilloscopes back to text ↑
- They were probably trained on videos found on the internet back to text ↑
- But somehow also twice as expensive to buy back to text ↑
- A big data labeling tech startup that I used to intern at back to text ↑